Beyond the route: Introducing granular MTA bus speed data
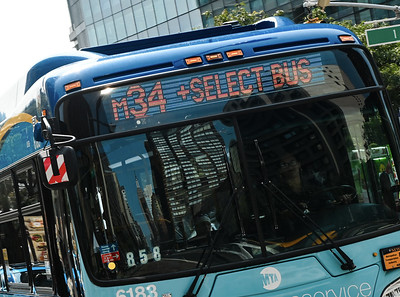
Beyond the route: Introducing granular MTA bus speed data
Updated September 24, 2024 9:00 a.m.
How fast are we moving?
Every weekday, the MTA runs over 4,900 buses across 327 routes, with around 1.3 million folks swiping or tapping to take a bus across the city. It is a remarkable feat, but even with that many passengers using our bus system, less is often written about our bus network than the subway. Given how vital our bus network is in providing travel to New Yorkers across the city, serving millions of residents, we at the MTA are always paying special attention to how this interconnected network can better serve riders.
Our buses, though, have to navigate a more complicated landscape than our trains, facing double-parked cars, road closures, delivery vehicles, traffic, and whatever else they might find on NYC’s frenetic roadways. Amidst this obstacle-laden environment, how fast are our buses actually going? Today, we are making it easier for the public to better understand this question with the new MTA Bus Route Segment Speeds Dataset on Open Data, which provides granular and in-depth bus speeds across our entire network.
Bus speeds between timepoints
The animation above shows this new Open Dataset visualized for an average Wednesday in May 2024, showing how fast buses are traveling between “timepoints” (i.e. the “major stops” on a bus route) for every route in the system. We can see how the general traffic congestion in Manhattan, Downtown Brooklyn, and the South Bronx leads to significant slowdown of bus speeds during the day—especially during morning and evening commutes. We can also see how, comparatively, routes in Eastern Queens and Staten Island travel much faster.
What is this data?
We utilize the GPS system on our buses to determine when each of our buses is at its major stops along a route. Combining this with the distance between each stop, we can then calculate how fast a bus travels between stops. (For more information about how the MTA uses “Dynamic Time Warping” to match GPS traces to bus routes, check out our previous blog post on “Bus Matching.”) Users of this data should note that these speed calculations include everything that a bus will encounter on its route—time the bus “dwells” to pick up passengers, stoplights, instances where buses change operators mid-route (“reliefs”), road closures, delivery vehicles, and traffic slowdowns—all of which are important for representing the true travel time of our customers.
Year |
Month |
Day of week |
Route ID |
Trip type |
Hour of day |
Timepoint stop name |
Next timepoint stop name |
Bus trips |
Speed |
---|---|---|---|---|---|---|---|---|---|
2024 |
5 |
1 |
B1 |
Local |
8 |
86 ST/ STILLWELL AV |
86 ST/18 AV |
24 |
6.19 |
2025 |
5 |
1 |
M103 |
Local |
8 |
3 AV/E 23 ST |
3 AV/ E 42 ST |
11 |
6.00 |
Looking at the data sample in Table 1, users will likely first ask, “What is a ‘timepoint’”? Many of our bus routes can have well over 50 stops, which can make managing and communicating about the route fairly difficult. For this reason, bus routes are divided up into what are known as “timepoints”, which are the major stops along a bus route. If you’ve ever looked at one the MTA’s bus schedules, like the M103 schedule shown in Figure 1, these are the stops that are displayed to the public along with expected arrival times. To keep the data at a workable size, it is these timepoint-to-timepoint bus speeds that are included in this new dataset.
Figure 1: M103 bus schedule
What can we do with this data?
By having this data at such a granular time and spatial level, users can dig much more deeply into how fast our buses are traveling across every pocket of the city.
Beginning at a high level, Figure 2 shows how average bus speeds change over the course of a typical Wednesday. We can see that buses move fastest during the overnight hours, before slowing significantly during the peak morning and afternoon hours. Figure 3 shows that, unfortunately, it is during these slow, congested hours that most of the MTA’s customers are riding.
Figure 2: City-wide weighted average Wednesday bus speeds between timepoints by hour
Figure 3: Average Wednesday paid ridership by hour
Digging deeper, though, we can use this dataset to begin investigating speeds along specific corridors of the city. Figure 4 shows a zoomed in view of how fast our buses travel north on 6 Avenue in Manhattan, between the 16 St and 34 St timepoints on the M7 bus route. We can see speeds for this bus reach its nadir around 12 p.m., running three times slower than its overnight speed, as heavy traffic reaches its midday peak.
Figure 4: Average weekday bus speed between timepoints by hour
This granular view allows transit planners at the MTA, and in the public, to investigate the slowest areas where our buses operate. (Figure 5 shows that during a weekday evening commute, for example, where our slowest road corridors show up througout the city.) We’ve also included in the dataset road distance, average travel time, and number of bus trips for every hour and timepoint pair so that users can calculate weighted averages across multiple routes if they choose.
While shifting bus speeds by one or two miles an hour may not sound too significant, over the course of an entire bus route it can represent the difference between a 40-minute and a 60-minute commute for a rider. Given the outsize effect this has on our riders, the MTA is always striving to develop new solutions (adding bus lanes, improving the boarding speeds, working with partners across fellow city and state agencies) to tackle this question of speed.
Figure 5: Slowest timepoints during evening commute
Go forth and query this data, with speed.
We are excited for Open Data users to dig into this dataset, experiment, and find insights from the “speed sample of NYC’s streets” that the MTA’s 4,900 buses collect each day. This data will be uploaded on a monthly basis, and can be found on the NYS Open Data portal.
So please explore, ask us questions, and if you build something cool with this data we would love to hear about it! Make your findings public and send us an email at [email protected].
About the authors
Jack Hui is a Senior Data Scientist on the Data & Analytics team. As a born and raised NYer, and a frequent user of the Staten Island Express Bus, he has strong opinions about bus speeds.
Matt Yarri is a Data Science Manager on the Data & Analytics team. He finds riding the M15 at night, on the open road, to be an exhilarating experience.