Cerebrum: What if we could recreate your brain?
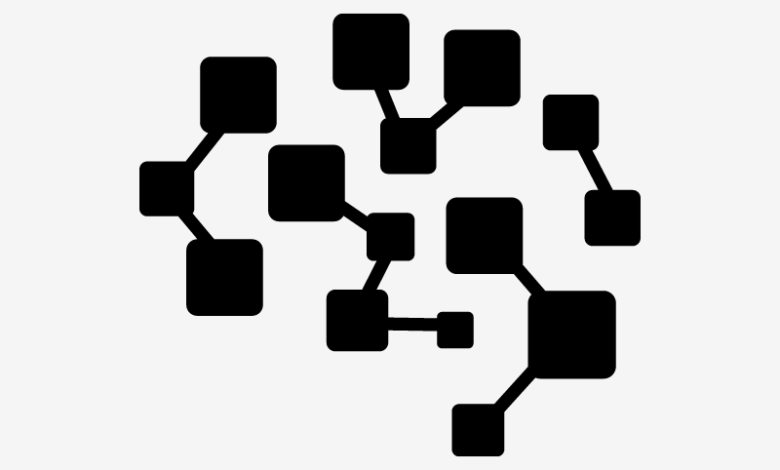
Introducing Cerebrum
What if we could simulate your brain?
Published: December 20th, 2024
By: Aarush Gupta
Paper: cerebrum.pdf
Email: [email protected]
Advancements in computational neuroscience are continually reshaping our understanding of the brain’s intricate networks. A key challenge in this field is deciphering the dynamic connectivity of neural networks, which is essential for both fundamental neuroscience and the development of clinical applications. To address this, we introduce Cerebrum, a novel framework that combines biologically inspired neuron models with cutting-edge machine learning techniques to simulate and infer synaptic connectivity in large-scale brain networks.
Bridging Biological Models and Machine Learning
Traditional approaches to studying brain networks often rely on graph theoretical methods that provide valuable insights into the static topological properties of neural connections. However, these methods typically overlook the temporal dynamics that are crucial for understanding how neuronal activity evolves over time. Cerebrum bridges this gap by integrating the Hodgkin-Huxley (HH) neuron model, known for its biological realism, with Graph Neural Networks (GNNs), which excel at learning complex patterns in graph-structured data. The HH model simulates the electrical characteristics of neurons, capturing the essential dynamics of action potentials and synaptic interactions. By combining this with GNNs, Cerebrum can effectively learn from simulated neuronal activity to predict the underlying synaptic connectivity. This integration allows for a more comprehensive analysis of brain networks, considering both their structural and dynamic properties.
To train and evaluate Cerebrum, we employed three canonical network topologies: Erdős-Rényi, Small-World, and Scale-Free. These topologies serve as ground-truth connectivity matrices, providing diverse structural frameworks for the GNNs to learn from. Each topology presents unique characteristics. Erdős-Rényi networks consist of randomly connected nodes, offering a baseline for connectivity without inherent clustering or hierarchy. Small-World networks feature high clustering and short path lengths, mimicking the efficient communication pathways observed in many biological networks. Scale-Free networks are characterized by the presence of hub nodes with a high degree of connectivity, reflecting the hierarchical organization seen in various neural systems. By training Cerebrum on these different topologies, we can assess its ability to generalize across diverse network structures and better understand the factors that influence connectivity inference.
To ensure that our simulations remain grounded in biological reality, Cerebrum incorporates empirical synaptic data from C. elegans, a model organism with a well-mapped neural connectome. By integrating real-world connectivity data, we enhance the biological fidelity of our simulations, allowing for more accurate and relevant predictions. This blend of synthetic and empirical data helps validate our approach and ensures that the insights gained are applicable to actual biological systems.
Incorporating Disease-Specific Dynamics
Cerebrum’s framework is designed to explore how pathological states affect neural network dynamics and connectivity. By introducing disease-specific parameter modifications into the HH neuron models, we can simulate conditions such as Parkinson’s disease and epilepsy. These modifications allow us to observe changes in neuronal firing patterns and synchronization, providing insights into how diseases disrupt normal brain function. This capability is particularly valuable for studying the impact of neurological disorders on large-scale brain networks. Understanding these disruptions at a network level can inform the development of targeted interventions and therapeutic strategies aimed at restoring healthy connectivity patterns.
Our comprehensive evaluations demonstrate that Scale-Free networks facilitate more accurate and robust connectivity inference compared to Erdős-Rényi and Small-World topologies. This superiority is attributed to the distinctive firing and synchronization dynamics inherent in Scale-Free networks, which provide clearer signals for the GNNs to learn from. Additionally, Scale-Free networks exhibit greater resilience to parameter variations, maintaining high prediction accuracy even under different conditions. Uncertainty estimation further confirms the reliability of Cerebrum’s predictions across all tested topologies, highlighting the robustness of the framework. These results underscore the potential of integrating biologically plausible models with advanced machine learning techniques to advance our understanding of brain connectivity.
Looking Forward
To facilitate further research and collaboration, we are releasing Cerebrum as an open-source computational toolkit. This toolkit provides tools for neural dynamics simulation, connectivity inference, and interactive network perturbations. By making Cerebrum accessible to the research community, we aim to encourage widespread adoption and collaborative improvement, accelerating progress in the field of computational neuroscience.
Cerebrum represents a significant step forward in modeling and analyzing large-scale brain networks. Moving forward, we plan to integrate real neural recordings with our framework, enhancing its applicability to in vivo studies. Additionally, expanding the range of disease-specific models will allow for a deeper exploration of various neurological conditions and their impact on brain connectivity. Collaboration with the broader scientific community will be crucial in refining Cerebrum and expanding its capabilities. By working together, we can push the boundaries of what is possible in understanding the brain’s complex networks and ultimately contribute to advancements in both neuroscience and clinical practice.
Cerebrum stands as a testament to the power of interdisciplinary approaches, combining biological realism with machine learning to tackle one of neuroscience’s most pressing challenges. We invite researchers and practitioners to explore the capabilities of Cerebrum and contribute to its ongoing development, driving forward our collective knowledge of the brain’s intricate connectivity.
Ad astra.