Why we need education-specific small language AI models
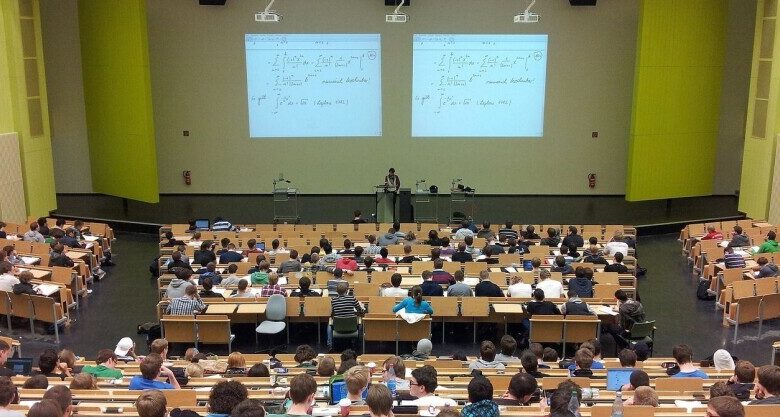
This article features an interview with Dan Rosensweig, who is speaking at TNW Conference on June 20. Check out the full event schedule here.
Artificial intelligence has the potential to transform education for the better. But when ChatGPT launched in 2022, it caused schools and academia alike to panic.
The AI model, able to reply to any question and write any text, was quickly denounced as a cheating-enabling tool — and one that undermined learning.
Fast-forward to 2024, panic has ceased and the education sector has recognised the potential of large language models (LLMs) to provide support to both students and teachers.
But to really align the potential of the technology with learning outcomes, what we need are education-specific small language models (SLMs), Dan Rosensweig, Executive Chairman at student learning platform Chegg, tells TNW.
The <3 of EU tech
The latest rumblings from the EU tech scene, a story from our wise ol’ founder Boris, and some questionable AI art. It’s free, every week, in your inbox. Sign up now!
“General purpose LLMs are a good place to start,” Rosensweig says. But they’re not specifically designed with students’ learning process in mind.
Such tools have proved that they can successfully consume vast amounts of data from the internet and summarise the information effectively. But they’re also prone to providing inaccurate answers and they even hallucinate.
“Generalist LLMs have no obligation to be accurate, or to be personalised, and they don’t know any individual student’s situation when it comes to what they know or what they don’t know,” Rosensweig says.
Conversely, small language models might have a more limited capacity to process information and generate text compared to LLMs, but they’re more efficient in meeting specific needs.
Education-specific SLMs require two elements, according to Rosensweig.
The first one is the incorporation of pedagogy by design. This refers to developing a model that “understands how students learn best, what their individual needs are, and that can assess the learning outcomes to move forward with the process.”
The second necessary element is a proprietary, accurate data set that is large enough to train AI across different subject verticals. In the case of Chegg, which has developed 26 SLMs, the data is based on over 100 million pieces of learning content, created over the past 10 years.
Rosensweig believes that the benefits of AI-powered personalised learning support are far-reaching.
“It starts with the learning process itself and then offers a blend between critical thinking and actual employable skills.”
Dan Rosensweig is one of many tech luminaries speaking at this year’s TNW Conference, which takes place on June 20-21 in Amsterdam. If you want to attend, we have a special offer for our readers. Use the code TNWXMEDIA at checkout to get 30% off your business pass, investor pass or startup packages (Bootstrap & Scaleup). We’re also offering a 50% discount for the Women in Tech ticket.